Using AI for Financial Analysis: 5 Effective Methods
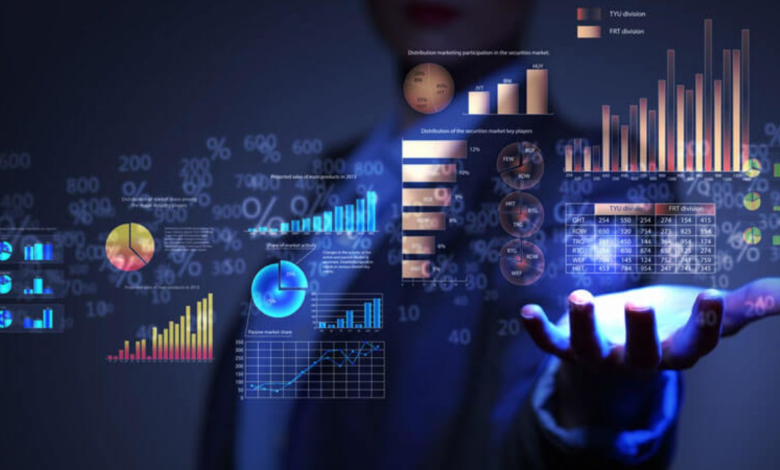
The financial sector has been an early and eager adopter of artificial intelligence (AI) technologies due to the massive datasets and analytical complexities inherent in markets, trading, transactions, risk modeling and beyond.
When properly implemented, AI techniques like machine learning and natural language processing can extract valuable insights from financial data at unprecedented speeds and accuracies to guide predictive modeling, investment decisions, portfolio optimization and fraud prevention.
This article will provide an overview of five proven approaches for effectively applying AI to transform financial analysis.
Natural Language Processing for Sentiment Analysis
Natural language processing (NLP) specialized for processing unstructured text data can help assess sentiment levels around financial topics and entities.
Definition of NLP in financial analysis
In this context, NLP refers to AI enabling understanding, interpretation and generation of human language related to finance. An advanced AI pdf reader can analyze word choice, context and tone within documents like financial reports, news, forum discussions and regulatory filings to gauge overall sentiment – positive, negative or neutral.
Application of NLP for analyzing sentiment in financial data
Banks, hedge funds and researchers apply NLP across financial texts to identify bullish or bearish sentiment levels that may impact markets. This informs trading strategies and models. As one example, analysis of earnings call transcripts using NLP may surface insights ahead of stock movements.
Machine Learning for Predictive Analytics
Increasingly, financial institutions are using machine learning algorithms to forecast various financial outcomes.
Overview of machine learning in financial analysis
Machine learning refers to AI systems that can learn from data patterns to make predictions without explicit programming. In finance, machine learning processes vast historical data to uncover relationships and make numeric forecasts like future prices or macroeconomic trends.
Methods for using machine learning for predicting financial outcomes
Banks may apply machine learning approaches like regression models or neural networks to predict customer credit risk, attrition and transactions based on past behaviors. Hedge funds might use AI to make market forecasts for commodities, bonds or currencies to inform trades.
Deep Learning for Time-Series Analysis
Advanced machine learning in the form of deep learning artificial neural networks excels at making sense of time-series data common in finance.
Explanation of deep learning in financial analysis
Deep learning refers to sophisticated AI models composed of multi-layered neural networks that can independently extract abstract features and patterns within time-series data. This handles complexity beyond human analysis.
Techniques for utilizing deep learning for time-series analysis in finance
Institutions can apply deep learning to financial time-series like historical pricing data to detect patterns for trading signals, risk analysis, volatility modeling and generating quantitative trading strategies optimized for changing markets.
Reinforcement Learning for Portfolio Optimization
Reinforcement learning has emerged as a technique for optimizing portfolios by determining data-driven buy/sell decisions and allocation.
Introduction to reinforcement learning in financial analysis
Reinforcement learning is a machine learning approach involving AI agents that can learn behaviors and improve actions through continuous trial-and-error interactions with dynamic environments like markets.
Strategies for portfolio optimization using reinforcement learning
As the AI agent makes simulated trades, it receives feedback on investment returns, allowing it to refine trading rules optimized for maximizing portfolio performance. This learned strategy can be operationalized to direct actual buy/sell order execution.
Computer Vision for Fraud Detection
Financial institutions also apply computer vision (AI processing pixel images) for enhanced fraud prevention.
Definition of computer vision in financial analysis
Computer vision is a subset of AI focused on enabling machines to identify, classify and understand objects within digital images and video through advanced pattern recognition and deep learning techniques.
Implementation of computer vision for fraud detection in financial transactions
Banks may leverage computer vision on customer transaction data to identify patterns indicative of fraudulent transactions vs legitimate ones based on factors like transaction type, location, merchant and more to block illegal purchases.
Conclusion
In summary, leading financial institutions are already using NLP for sentiment analysis, machine learning for predictive analytics, deep learning for time series modeling, reinforcement learning for portfolio optimization and computer vision for fraud – achieving previously impossible performance levels.
As computing power grows exponentially, financial analysis utilizing AI will become faster, more predictive and hyper personalized down to the customer level – transforming legacy processes through previously unthinkable automation.